You Now About Tvalue.
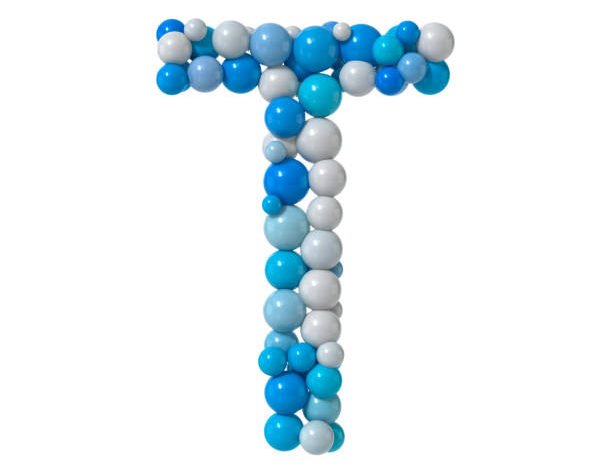
The term “t-value” is a critical concept in statistics, often used in hypothesis testing and data analysis. Whether you are a student, a researcher, or a professional in a data-driven field, understanding the t-value is essential for interpreting statistical results effectively. This article delves into the definition, significance, calculation, and practical applications of the t-value, providing a comprehensive overview for anyone seeking clarity on this fundamental statistical metric. You now about tvalue.
What is T-Value?
The t-value, also known as the test statistic, is a ratio that compares the difference between an observed sample statistic and its hypothesized population parameter against the variability of the sample. Essentially, it helps determine whether the difference observed in a dataset is significant or merely due to random chance. The t-value is a cornerstone of the Student’s t-test, a statistical test used to assess hypotheses about population means.
Importance of the T-Value
The t-value plays a crucial role in hypothesis testing. In statistics, researchers often begin with a null hypothesis, which assumes no effect or no difference between groups. By calculating the t-value, one can evaluate the likelihood of observing the sample data if the null hypothesis were true. A higher absolute t-value indicates a greater departure from the null hypothesis, suggesting that the observed effect may be statistically significant.
Calculating the T-Value
The formula for calculating the t-value depends on the type of t-test being performed. However, the general formula is:
Where:
- t is the t-value.
- is the sample mean.
- is the population mean under the null hypothesis.
- s is the standard deviation of the sample.
- n is the sample size.
The formula essentially quantifies how far the sample mean deviates from the hypothesized population mean, adjusted for sample variability and size.
Types of T-Tests
- One-Sample T-Test: Compares the mean of a single sample to a known population mean.
- Independent Two-Sample T-Test: Evaluates the difference between the means of two independent groups.
- Paired T-Test: Compares the means of two related groups or repeated measurements.
Each type of t-test has slightly different assumptions and applications but relies on the same fundamental principles of t-value calculation.
Interpreting the T-Value
Interpreting the t-value requires comparing it to a critical value from the t-distribution, which varies based on the sample size (degrees of freedom) and the chosen significance level (e.g., 0.05). If the absolute t-value exceeds the critical value, the null hypothesis is rejected, indicating that the observed effect is statistically significant.
P-Value and T-Value
The p-value complements the t-value by providing the probability of obtaining a test statistic as extreme as the observed value, assuming the null hypothesis is true. A low p-value (typically less than 0.05) suggests strong evidence against the null hypothesis, aligning with a high t-value.
Practical Applications of the T-Value
1. Scientific Research
T-tests are widely used in scientific experiments to determine whether experimental interventions have significant effects. For example, a researcher might use a t-test to compare the test scores of students taught using two different teaching methods.
2. Medicine and Healthcare
In clinical trials, t-tests help evaluate the efficacy of new treatments or drugs. By comparing outcomes between treatment and control groups, researchers can determine whether observed differences are statistically significant.
3. Business and Marketing
Businesses often use t-tests to analyze consumer behavior, assess the effectiveness of marketing strategies, or optimize pricing models. For instance, a company might compare sales figures before and after a promotional campaign to evaluate its impact.
4. Quality Control and Manufacturing
Manufacturers employ t-tests to monitor product quality and consistency. By comparing sample measurements against desired specifications, they can identify potential deviations or defects.
Limitations of the T-Value
While the t-value is a powerful statistical tool, it is not without limitations. Key challenges include:
- Sensitivity to Sample Size: Small sample sizes can lead to unreliable t-values, as variability increases with fewer data points. Large samples, on the other hand, may detect trivial differences as significant.
- Assumptions of Normality: T-tests assume that the data are normally distributed. Violations of this assumption can affect the accuracy of the t-value.
- Independence of Observations: The validity of the t-value depends on the independence of observations. In cases of non-independence, specialized statistical methods are required.
Enhancing Understanding Through Visualization
Visual aids such as graphs and plots can make the concept of the t-value more accessible. For instance, plotting sample means and confidence intervals can help illustrate how the t-value quantifies differences relative to variability.
Conclusion
The t-value is an indispensable tool in statistics, enabling researchers and analysts to draw meaningful conclusions from data. By understanding its calculation, interpretation, and applications, you can effectively use the t-value to test hypotheses, validate assumptions, and make data-driven decisions. However, it is equally important to recognize its limitations and apply it judiciously in appropriate contexts. Mastery of the t-value not only enhances statistical literacy but also empowers individuals to unlock deeper insights from data in various fields.